import wandb
from datasets import load_dataset
# if you can't find libwebp library, use brew update && brew install webp
import evaluate
import numpy as np
import torch
from transformers import TrainingArguments
from trl import SFTTrainer
from transformers import (
AutoModelForCausalLM, AutoTokenizer, AutoConfig,
LlamaConfig, LlamaModel,
)
from transformers import GenerationConfig
from transformers.integrations import WandbCallback
from tqdm import tqdm
def token_accuracy(eval_preds):
token_acc_module = evaluate.load("accuracy")
logits, labels = eval_preds
# shape: batch_size x max_sequence_length
predictions = np.argmax(logits, axis=-1)
# accuracy only accepts 1d array. So if the batch contains > 1 datapoints,
# the accuracy is based on flattened arrays
# https://huggingface.co/spaces/evaluate-metric/accuracy
return token_acc_module.compute(
predictions=predictions.flatten().astype(np.int32),
references=labels.flatten().astype(np.int32),
)
def prompt_no_input(row):
return ("Below is an instruction that describes a task. "
"Write a response that appropriately completes the request.\n\n"
"### Instruction:\n{instruction}\n\n### Response:\n{"
"output}").format_map(
row
)
def prompt_input(row):
return (
"Below is an instruction that describes a task, paired with an input "
"that provides further context. "
"Write a response that appropriately completes the request.\n\n"
"### Instruction:\n{instruction}\n\n### Input:\n{input}\n\n### "
"Response:\n{output}").format_map(
row
)
def create_alpaca_prompt(row):
return prompt_no_input(row) if row["input"] == "" else prompt_input(row)
class LLMSampleCB(WandbCallback):
def __init__(
self, trainer, test_dataset, num_samples=10, max_new_tokens=256,
log_model="checkpoint"
):
super().__init__()
self._log_model = log_model
def create_prompt_no_anwer(row):
row["output"] = ""
return {"text": create_alpaca_prompt(row)}
self.sample_dataset = test_dataset.select(range(num_samples)).map(
create_prompt_no_anwer
)
self.model, self.tokenizer = trainer.model, trainer.tokenizer
self.gen_config = GenerationConfig.from_pretrained(
trainer.model.name_or_path,
max_new_tokens=max_new_tokens
)
def generate(self, prompt):
tokenized_prompt = self.tokenizer(prompt, return_tensors='pt')[
'input_ids']
with torch.inference_mode():
output = self.model.generate(
inputs=tokenized_prompt, generation_config=self.gen_config
)
return self.tokenizer.decode(
output[0][len(tokenized_prompt[0]):], skip_special_tokens=True
)
def samples_table(self, examples):
records_table = wandb.Table(
columns=["prompt", "generation"] + list(
self.gen_config.to_dict().keys()
)
)
for example in tqdm(examples):
prompt = example["text"]
generation = self.generate(prompt=prompt)
records_table.add_data(
prompt, generation, *list(self.gen_config.to_dict().values())
)
return records_table
def on_evaluate(self, args, state, control, **kwargs):
super().on_evaluate(args, state, control, **kwargs)
records_table = self.samples_table(self.sample_dataset)
self._wandb.log({"sample_predictions": records_table})
print("log once")
def param_count(m):
params = sum([p.numel() for p in m.parameters()]) / 1_000_000
trainable_params = sum(
[p.numel() for p in m.parameters() if p.requires_grad]
) / 1_000_000
print(f"Total params: {params:.2f}M, Trainable: {trainable_params:.2f}M")
return params, trainable_params
def trl_train():
wandb.login(key='replace_with_your_own')
lr = 2e-5
batch_size = 8
max_steps = 4
# evaluate every eval_steps. so if we set max_steps = 4 and
# eval_steps = 2, we will evaluate twice during training
eval_steps = 2
num_eval_data = 5
num_wandb_cb_eval_data = 7
wandb_cb_max_new_tokens = 256
num_train_epochs = 1
max_seq_length = 1024
gradient_accumulation_steps = 1
gradient_checkpointing = False
output_dir = "./output/"
run = wandb.init(
project="second_project",
config={
"lr": lr,
"batch_size": batch_size,
"max_steps": max_steps,
"eval_steps": eval_steps,
"num_eval_data": num_eval_data,
"num_wandb_cb_eval_data": num_wandb_cb_eval_data,
},
)
alpaca_ds = load_dataset("winglian/alpaca-gpt4-split")
train_dataset = alpaca_ds["train"]
eval_dataset = alpaca_ds["test"]
model_id = 'meta-llama/Llama-2-7b-hf'
# try different ways to initialize a llama model
# method 1: construct LLamaModel from LlamaConfig
# https://huggingface.co/docs/transformers/v4.37.2/en/model_doc
# /llama2#transformers.LlamaConfig
# configuration = LlamaConfig(
# num_hidden_layers=2,
# hidden_size=32,
# intermediate_size=2,
# num_attention_heads=1,
# num_key_value_heads=1,
# )
# model = LlamaModel(configuration)
# param_count(model)
# method 2 & 3 need to wait for token approval
# https://huggingface.co/meta-llama/Llama-2-7b-hf
# method 2: load config first, tune down model size, then initialize the actual LLM
# https://discuss.huggingface.co/t/can-i-pretrain-llama-from
# -scratch/37821/8
config = AutoConfig.from_pretrained(model_id)
config.num_hidden_layers = 1
config.hidden_size = 2
config.intermediate_size = 2
config.num_attention_heads = 1
config.num_key_value_heads = 1
model = AutoModelForCausalLM.from_config(config)
param_count(model)
# method 3: directly load pretrained llama model, which may encounter OOM
# on a consumer cpu machine
# model = AutoModelForCausalLM.from_pretrained(
# model_id,
# device_map="auto",
# trust_remote_code=True,
# low_cpu_mem_usage=True,
# torch_dtype=torch.bfloat16,
# load_in_8bit=True,
# )
training_args = TrainingArguments(
output_dir=output_dir,
use_cpu=True,
report_to="wandb",
per_device_train_batch_size=batch_size,
bf16=True,
learning_rate=lr,
lr_scheduler_type="cosine",
warmup_ratio=0.1,
max_steps=max_steps,
eval_steps=eval_steps,
num_train_epochs=num_train_epochs,
gradient_accumulation_steps=gradient_accumulation_steps,
gradient_checkpointing=gradient_checkpointing,
gradient_checkpointing_kwargs={"use_reentrant": False},
evaluation_strategy="steps",
# logging strategies
logging_strategy="steps",
logging_steps=1,
save_strategy="no",
)
tokenizer = AutoTokenizer.from_pretrained(model_id)
tokenizer.pad_token = tokenizer.eos_token
trainer = SFTTrainer(
model,
tokenizer=tokenizer,
args=training_args,
train_dataset=train_dataset,
eval_dataset=eval_dataset.select(list(range(num_eval_data))),
# this tells the trainer to pack sequences of `max_seq_length`
# see illustration in https://wandb.ai/capecape/alpaca_ft/reports/How
# -to-Fine-tune-an-LLM-Part-3-The-HuggingFace-Trainer--Vmlldzo1OTEyNjMy
packing=True,
max_seq_length=max_seq_length,
formatting_func=create_alpaca_prompt,
compute_metrics=token_accuracy, # only call at evaluation
)
wandb_callback = LLMSampleCB(
trainer, eval_dataset,
num_samples=num_wandb_cb_eval_data,
max_new_tokens=wandb_cb_max_new_tokens,
)
trainer.add_callback(wandb_callback)
trainer.train()
wandb.finish()
# other materials:
# fine tune ppo vs dpo
# trl stackllama tutorial:
# https://huggingface.co/docs/trl/using_llama_models
# trl readme: https://github.com/huggingface/trl/tree/main?tab=readme-ov
# dpo - trl: https://huggingface.co/blog/dpo-trl
if __name__ == '__main__':
trl_train()
Now let’s examine the code in more details:
First, we initialize a weights & bias project (wandb.init(...)
), which is used for logging intermediate training/evaluation results. It is a very convenient tool for logging and visualization.
Then, we use load_dataset(...)
, an api from HuggingFace’s dataset library, to load a specific data. HuggingFace hosts many awesome datasets at https://huggingface.co/datasets.
Next, we initialize an actual LLM. Since this is a minimal example, I created a tiny LLM by modifying its config to have very few hidden layers and hidden sizes.
Next, we initialize TrainingArguments. We may need to be familiar with several concepts in TrainingArguments, such as gradient accumulation.
We then initialize a tokenizer, which is trivial by calling HuggingFace’s API AutoTokenizer.from_pretrained(...)
.
We then initialize SFTTrainer
, the main class for training and evaluating the LLM. Setting packing=True
means that we pack multiple individual sequences into a fixed-length sequence so that we can avoid much padding. Individual sequences are usually separated with an eos
token.
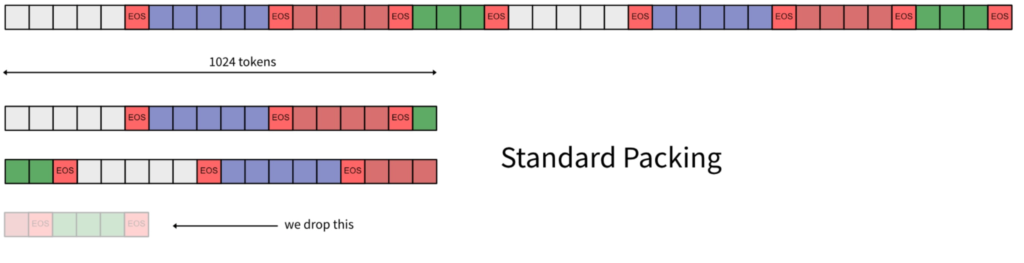
We also initialize a callback, which is called only in the evaluation stage. The callback class needs to first remove output in the dataset for evaluation.
We now look at the results logged in wandb (example https://wandb.ai/czxttkl/third_project/runs/hinck0h5):
- Since we specify
max_steps=4
and eval_steps=2
, we have 2 evaluations. The evaluation loss curves verifie we indeed log 2 evaluation results.

- we have a table showing the results from the callback. We can verify that prompts indeed have outputs removed. We can also use
runs.history.concat["sample_predictions"]
instead of runs.summary["sample_predictions"]
to check the evaluation results from all evaluation runs (exactly 2 runs) (see the reference in https://wandb.ai/morg/append-to-table/reports/Append-to-Table–Vmlldzo0MjY0MDIx)
